From signal processing to quantum entanglement, Shayan Garani’s interests encompass a diverse range of complex problems
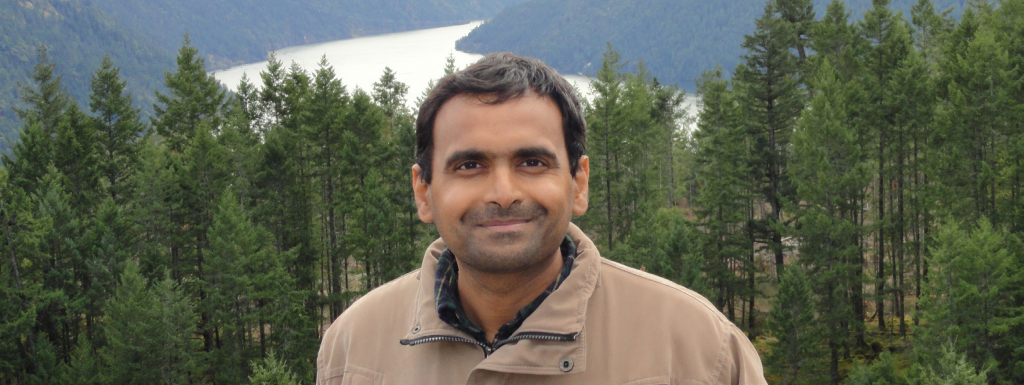
“The cube is a sort of [quantum computer] memory, where the vertices are qubits, and the faces are stabilisers,” says Shayan Srinivasa Garani, Associate Professor at the Department of Electronic Systems Engineering, when asked about the inspiration for his lab logo. Sitting across a large wooden desk stacked with papers, patents, and awards for research in diverse fields, he adds, “A coded quantum memory has been my dream.”
The Physical Nano-memories, Signal and Information processing Laboratory (PNSIL), led by Shayan works on solving problems in diverse areas, from signal processing and machine learning to tackling quantum information problems.
A major focus of PNSIL since it was set up has been solving problems in error correction. Information systems and signals in modern electronic devices are prone to errors during transmission and storage. To overcome these errors, computer scientists devised techniques in which by replacing certain symbols of information with other (and a larger number of) symbols, the signals can be corrected, making them robust against errors. The set of symbols are called codewords and this process of replacement is called encoding. Different encoding schemes have different properties and advantages. One of PNSIL’s main efforts is to study and analyse the mathematical properties of these codes, design better codes for various applications, and design efficient encoding and decoding architectures.
Shayan’s tryst with coding theory goes back many years before he started PNSIL. After completing his Master’s degree at the University of Florida, he briefly worked in a telecommunication company before undertaking his doctoral studies at the Georgia Institute of Technology. A significant chunk of his doctoral research involved studying and solving problems related to non-binary 2D constrained codes. When he joined the industry after his PhD, he was involved in developing what are called Low-Density Parity Check (LDPC) codes for deployment in magnetic hard disks and solid-state drives.
LDPC codes are also known as Gallager codes, named after Robert G Gallager, who developed them in 1960. LDPC codes are known to be capacity-achieving, which means that the speed of information transfer in some transmission channels – like an optical fibre – is as fast as mathematically predicted for that channel.
LDPC codes have been well-studied since their introduction. Shayan was initially disinterested in them as he felt that most problems were already solved. It was only when his advisor and colleagues pointed out various problems in their practical implementation and the many puzzles yet to be solved that he was intrigued once again. Now he considers LDPC codes as one of his favourite classical coding schemes. In recent years, his lab has published various studies on hardware implementations of LDPC codes, developed 2D LDPC codes along with efficient 2D signal processing algorithms for next-generation storage systems. He is also venturing into LDPC codes for the transmission of quantum information.
When Shayan joined IISc, he was keen on pursuing the many puzzles in quantum information research, an area that had fascinated him for many years. He was first exposed to quantum information at a talk by Umesh Vazirani, a renowned computer scientist, in 2004. Quantum information technologies promise secure communication systems and speedups in solving various problems that are considered ‘tough’ to solve with classical computers. Quantum technologies are now just behind the horizon, and PNSIL works on various problems and new algorithms that assist in making these technologies a reality.
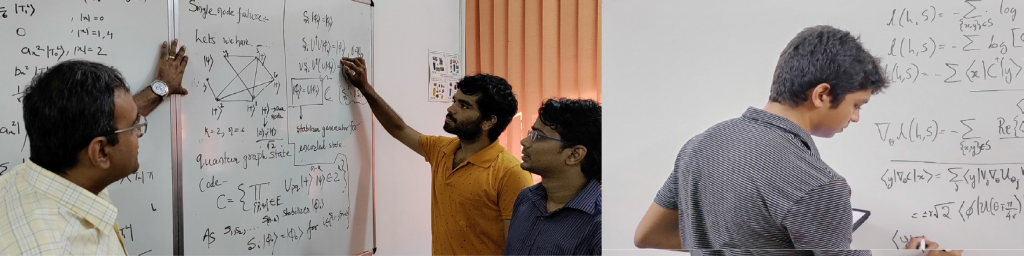
Entanglement also fascinates Shayan. It is a phenomenon where two physically separate entities, like atoms or photons, become linked even if they are very far apart, and inexplicably start influencing each other’s behaviour at the quantum level. Such far-flung connections could open up the possibility of quick and seamless transmission of both classical and quantum information over long distances.
A quantum channel, like an optical fibre, is used to transmit quantum information. As a coding theoretician, it was natural for him to explore the potential of entanglement in designing codewords for the transmission of quantum information. To reduce errors, this information is encoded using quantum error correction codes (QECCs), similar to classical error correction. His lab has developed comprehensive theoretical frameworks and efficient encoding architectures for entanglement-assisted versions of QECCs. Shayan and his students have also proposed novel methods to encode quantum information distributed amongst multiple users and recover lost information. He has also begun exploring and tackling problems in quantum neural networks, combining his interests in machine learning and quantum information.
Another major focus of the lab is solving theoretical problems in machine learning and neural networks, particularly in mimicking the way in which the human brain stores memories, building upon results from his Master’s thesis.
“Have you noticed that when you recite poems, learn slokas, or even prove theorems, you may [initially] have a missing link? But when you get the missing link, boom! You suddenly remember,” he explains. Called temporal plasticity, this ability to store and recall patterns, helps us anticipate what comes next depending on what information is already stored in our brain. Shayan explains this using an analogy: Imagine that you are in a kitchen. There are cooking tools and there are vegetables. Both are related and there is a sort of clustering in your brain of the items based on their interrelations. When you are cooking, there is a sequence of actions and items involved, and over time, this clustering evolves as the actions are performed. Earlier, there were well-established frameworks only for spatial clustering. Shayan’s lab has provided the necessary theoretical framework to understand the temporal evolution of this information in the brain and implemented these schemes in the form of neural networks on computers.
Aside from his research and teaching, Shayan tends to his garden in his free time. He is also a trained and practising Carnatic vocalist, and sometimes has jamming sessions with his students. It is of no surprise, therefore, that he has worked on music theory and processing too – he has presented papers on related topics at conferences. His lab has been exploring fundamental questions like what is music? What is a raaga? Should music be thought of as discrete or continuous? How can sound waveforms and gamakams be synthesised? He hopes that his contributions will help musicians better understand and analyse music.
Shayan says that he has always been very curious about every field. “You must be drawn to hard problems and have the endurance to work on them,” he says when asked about what students should focus on. Having a lab with diverse students – from undergraduates to postdocs who have studied distinctive fields like physics, electrical engineering, and mathematics – has enabled him to work on a broad spectrum of problems that have piqued his interest.
“You can have many ideas. Still, unless you have a group of students to work with and get solutions on paper, they are just going to remain in your mind,” he adds. “Nothing can beat that smile on your face when you solve a puzzle, and it is laid out correctly and is picture-perfect, and you can claim that no one else in the world has done that, [but] you’ve done that.”
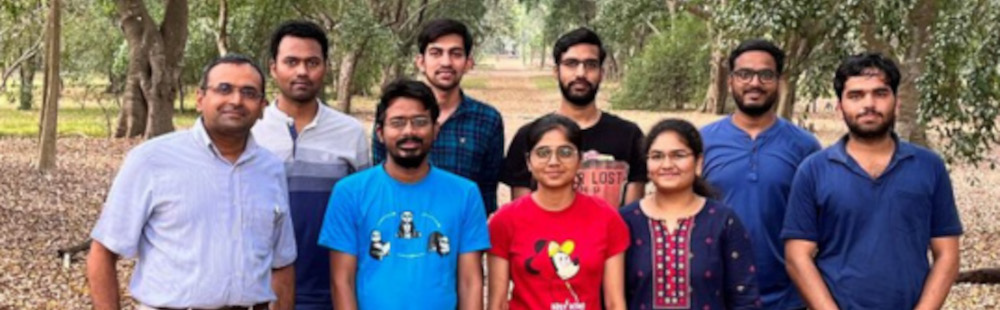