Inspired by the human brain, researchers at IISc and elsewhere are trying to make smarter and faster computing devices
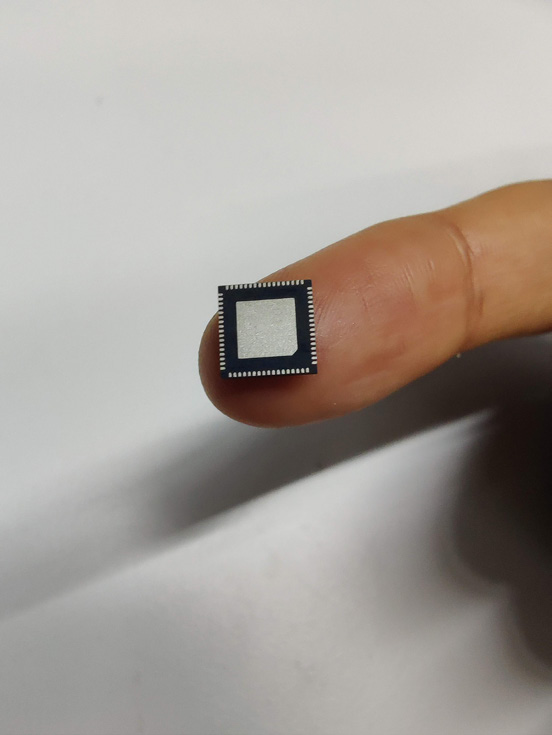
(Photo: NeuRonICS lab)
Consider a child playing on a swing, while licking on a candy, waving to her mom, and joining the other kids in a song from their favourite cartoon show. It may not seem extraordinary, but look closely and you will see that a four-year old is carrying out complex coordinated body movements, visual search, social engagement and memory recall, all at the same time. The best of our robots, in contrast, can only do a half-decent job at one of these tasks. Our brains clearly outsmart computers. Heck, even chicken brains outsmart our best computers.
The realisation of such limits has led to the rise of ‘neuromorphic’ computing. At its core lies inspiration from biological systems because the “nervous system is the most sophisticated system that engineers can learn from, which is … developed over billions of years of evolutionary process,” says Chetan Singh Thakur, Assistant Professor at IISc’s Department of Electronic Systems Engineering (DESE). The features that make our brains super-efficient, he explains, are the ‘PPA’ – power, performance and area metrics – a term routinely used by circuit designers.
Weighing just 1.5 kg, the human brain is compact, highly efficient and power-thrifty (the calories in a banana can keep it energised. Contrast that with a desktop computer which uses up to 250 watts – the equivalent of 2,000 bananas an hour). In addition, our brains are fault-tolerant, as they can adapt to neuron loss, and are self-learning. Neuromorphic engineering aims to marry these features, essentially etching brain architecture on to solid state devices.
Neuromorphic computing combines diverse disciplines – biology, electrophysiology, signal processing and circuit design, to name a few. In 2015, such interdisciplinary exchange at IISc led to the formation of the Brain, Computation and Data Science initiative, supported by the Pratiksha Trust. The group brings together labs from DESE, Centre for Neuroscience (CNS), Molecular Biophysics Unit (MBU), Electrical Communication Engineering (ECE) and other departments. An interdisciplinary PhD programme has been launched under its umbrella, along with three Distinguished Chair positions.
One of the groups under this initiative is the NeuRonICS lab, headed by Thakur, where research spans diverse levels. On one end, they work on algorithms for “event-based sensors”, which are sensory devices modelled on biological systems. An example of this is a neuromorphic camera, which can be more data- and power-efficient than conventional cameras. Its speedy tracking makes it useful in applications such as recognising high-speed activity and detecting anomalies. The lab is also developing algorithms for a ‘silicon cochlea’, an FPGA-based model of the cochlear system that can act as an efficient pre-processing unit for speech-based machine learning applications (FPGA or Field-programmable gate array is an integrated circuit that can be programmed after manufacturing for different applications).
Moving up from such sensors, the lab has also successfully modelled a network of grid cells and place cells – types of neurons that help us track where we are. This recent work is a collaboration with Rishikesh Narayanan, Associate Professor at MBU. This spatial navigation model could be used by robots to move intelligently in novel environments. Such ‘biomimetic’ systems can be built on silicon chips, another area of focus in the NeuRonICS lab. Towards this goal, they have used novel devices to model neuronal interconnections (synapses).
In contrast to Thakur’s approach, Bharadwaj Amrutur, Chair of the Robert Bosch Centre for Cyber Physical Systems and Sujit K Sikdar, Professor at MBU, have been working on developing neuro-electric hybrid devices by using neurons grown in the lab rather than recreating them in silicon. This brain-in-a-dish approach harnesses the parallel computing capacity and power efficiency of neurons as well as the accessibility of well-developed hardware and software.
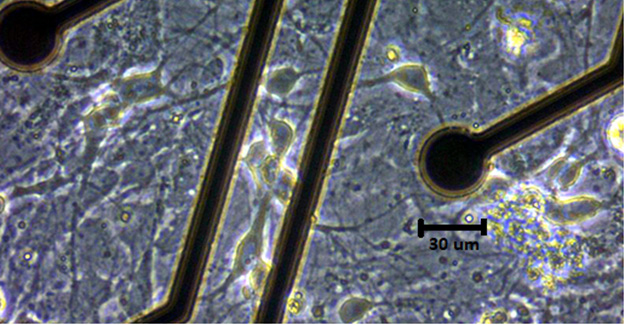
(Photo: Grace Mathew Abraham)
In an earlier study, they connected input devices (sensors) to a live neuronal culture which was specially grown on metal contacts called electrodes. The electrodes conducted appropriate signals from sensors to neurons to compute a solution for an obstacle avoidance problem. The resulting activity of the neurons was used to control a navigating robot seamlessly in real time.
In another project, they used similar neuronal cultures to demonstrate memory traces. They trained the network using a “barrage of inputs” so that specific connections developed between neurons. Despite these disturbances, the network did not go into overdrive, and remained stable – a condition called global homeostasis. This work might lead to better understanding of learning and memory, which require persistent input-driven changes in neuronal networks while maintaining stability. In collaboration with Thakur’s lab, the group is also currently working on interfacing a neuromorphic cochlea with a live neuronal culture to understand how a neuronal network distinguishes sound signals.
Neuromorphic computing is a young but rapidly growing field, with huge challenges ahead. How one neuron can have thousands of synapses in a miniscule space is a ‘routing’ problem yet to be solved in silicon, as denser connections can lead to dissipated currents, wrecking the whole enterprise. Another challenging area is communication. Neurons compute on a gradation of inputs, but send a spike as output – hence banking on both continuous and discrete signals. Neuromorphic design too will have to incorporate mixed signal processing, to perform efficient communication along with computation.
Better neuromorphic designs can lead to developing large-scale neural chips to simulate brain areas, and ask “better questions” about the brain – something Thakur looks forward to doing. He sees this as coming full circle with the two goals that early neuromorphic researchers had – the engineering goal of building neuro-inspired systems, and the scientific goal of using them to study brains. Successfully building a brain-like network in silicon could result in a computing resource for neuroscientists, enabling them to perform some experiments without having to use animals.
As we navigate these technical challenges, neuromorphic computing raises new questions in the fields of science policy, law and ethics. And at the other end of such challenges is Thakur’s question: “Can we build something that is useful?”