Rajiv Soundararajan’s lab is working on futuristic technologies that enable superior visual experiences
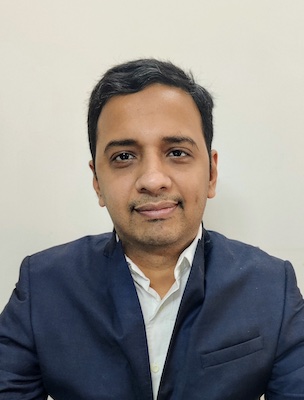
In today’s Instagram age, we are bombarded with images and videos every second of our day. But not every Instagrammer can be an ace photographer. Photos and videos can sometimes be blurred or unfocused, their quality might get reduced due to compression, the camera might shake. How do you check and improve the quality of such not-so-perfect images and videos? It is this question that drives the research in the lab of Rajiv Soundararajan, Associate Professor at the Department of Electrical and Communication Engineering. His lab is developing tools to assess the quality of images and videos.
Years ago, during his undergraduate days at BITS Pilani, Rajiv became intrigued by visual media. This led him to do a PhD at the University of Texas at Austin with faculty members who were experts in information theory, and image and video quality assessment. During his PhD, he worked on building benchmark datasets and algorithms for image quality assessment.
He created a database of high-quality reference videos and performed operations such as compression to distort the videos. Then, he designed experiments in which human subjects scored each video in the database for its quality. The results from these experiments became the first publicly available repository of labelled, human-scored videos. In the process, Rajiv and his collaborators learnt a lot about the different types of distortions in videos and images. They realised something important – that they could use image statistics to assess image quality and assign scores without involving humans. The algorithms based on this concept, called ‘blind image quality assessment’, were developed around 2010 and have been doing well in datasets generated years later. They also developed computer models called ‘natural scene statistics’ models, which capture the statistical regularities of natural images, such as similar intensities in nearby pixels.
Natural scene statistics models are based on the duality principle: the human visual system has evolved to match what is in the real world. “So if you are modelling what is out there, in some sense, you are implicitly modelling human perception of images. Sometimes it’s a humbling experience when you think some idea would work, but actual human opinions turn out to be very different,” says Rajiv.
For his work on these algorithms, Rajiv received the IEEE Best Paper Award for Circuits and Systems for Video Technology in 2016, and for Signal Processing in 2017. Significantly, their work also led to him and his collaborators receiving an Emmy Award from the National Academy of Television Arts and Sciences, USA, in 2021 for the “Development of Perceptual Metrics for Video Encoding Optimisation.” Video streaming platforms like Netflix leverage their algorithms to compress videos efficiently while maintaining optimal viewing quality for the human eye. By incorporating principles from visual neuroscience, the algorithms ensure a visually pleasing experience even with compressed files. “It did feel really good to be recognised for these efforts,” reflects Rajiv.
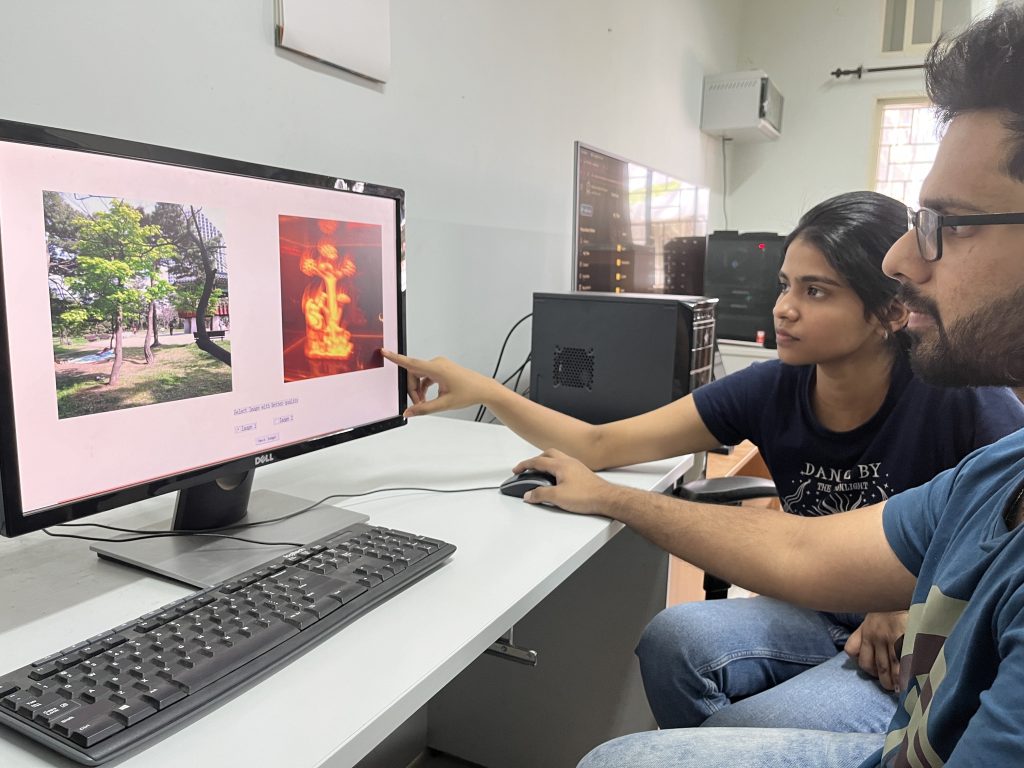
After his PhD, he worked at Qualcomm research on designing chipsets for various steps in a pipeline that processed images captured by the camera. These chipsets in the camera pipeline enable image correction such as noise reduction, lens shading correction, enhancing the image quality, and so on. “[But] I felt restricted in the industry working on just a single camera pipeline and a single device,” Rajiv says. “I wanted to do something that would be more all-pervasive, and I decided to switch back to academia.”
Evolving images and distortions
Rajiv’s lab at IISc, which he started in 2015, has continued to focus on image quality assessment.
In blind image quality assessment, there is no reference to a ‘pristine’ image to compare the distorted image. This contrasts with ‘full-reference quality assessment’ techniques used earlier in which the distorted image was compared with an original ‘pristine’ image, and machine learning models were trained on human-assigned scores. However, with the increasing use of mobile phone cameras from the 2010s, there are no original reference images to compare the distorted images, and the concept of ‘no reference image quality assessment’ has become more important. Camera systems continue to evolve, as do the kinds of images generated and the distortions associated with them. For example, panoramic screens that display 360-degree views are susceptible to new types of image artefacts (distortions) because multiple images are stitched together to create the panoramic view.
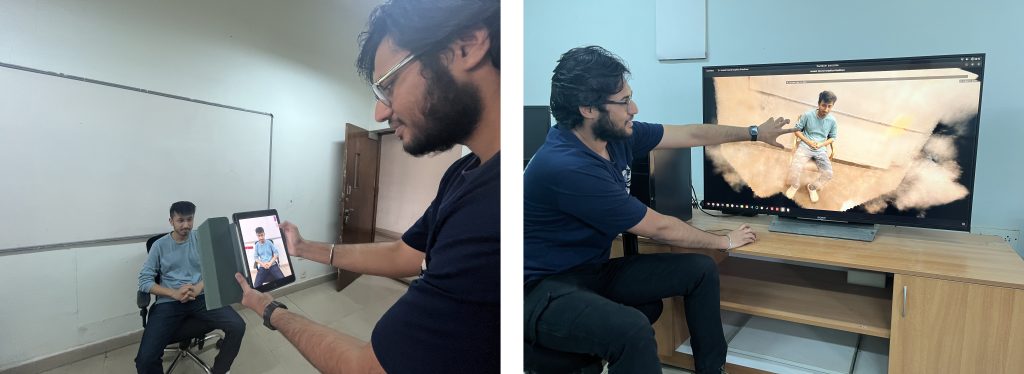
The rise of AI-generated images is also a challenge. AI-generated images and the types of distortions associated with them are very different from what have been encountered before.
As newer images and distortions emerge, computer models that are trained on an older database might not work well for new applications. “Our lab is currently looking at how to address this problem, and we are working on ‘generalisability’ of quality assessment tools,” explains Rajiv. One approach they use, for example, involves adapting a previously trained model using target-specific data. Another involves ‘semi-supervised learning’, where models are trained on small amounts of labelled data and large amounts of unlabelled data.
His lab is also working on novel view synthesis and image reconstruction. While watching a football game, there are a few cameras from some points of view. For a more enriching and realistic viewer experience, we would want to generate views from different points of view. Generating novel views can also be used in telepresence to view the local environment of the person on the other side of a video call. Current methods, including the popular ‘neural radiance field’ method need many input views. However, some applications only provide limited inputs. So, his group is now working on training models that work well even with very few inputs and images.
Rajiv’s ultimate goal is to enable superior visual experiences by working with evolving technologies and devices – imagine watching the thundering crowd at a Wimbledon match while sitting on your couch or exploring the Giza pyramids through a Virtual Reality headset.
The fact that some of the methods his lab has developed are being used for many real-world applications is very satisfying, Rajiv says. “Awards come as a result of that.” His advice to students is also along the same lines: “Be passionate about something that you want to solve. Try to solve the problem because you want to solve it, and not in expectation of rewards.”
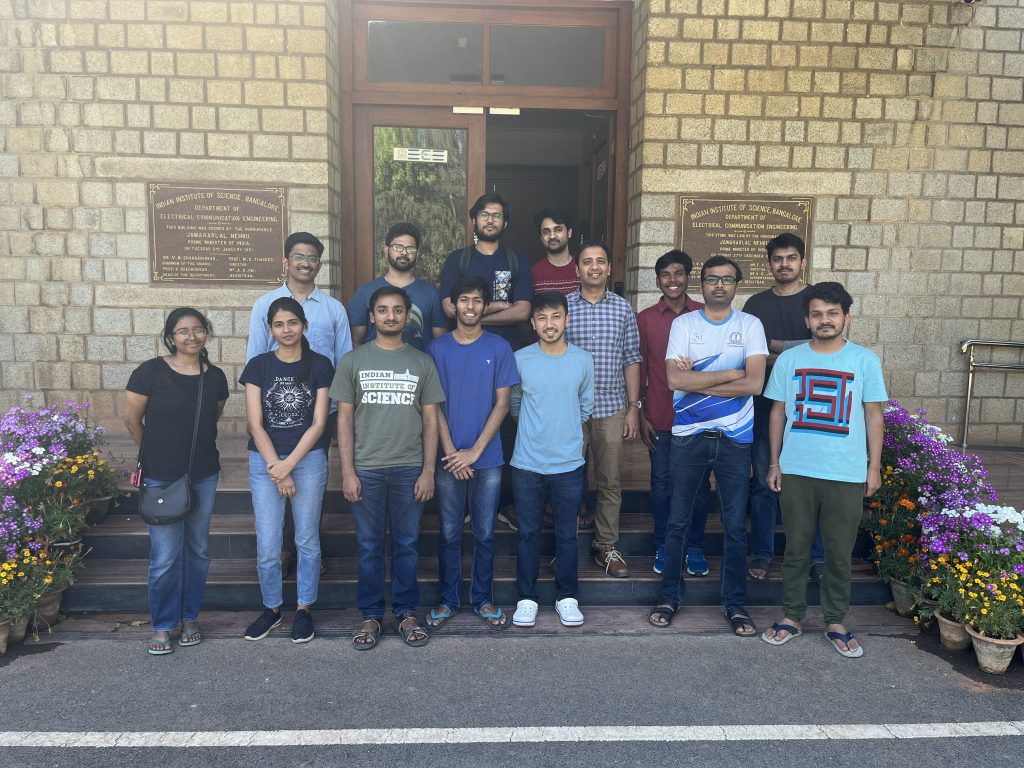