Garima Jindal’s lab uses computational tools to understand and design more efficient catalytic reactions
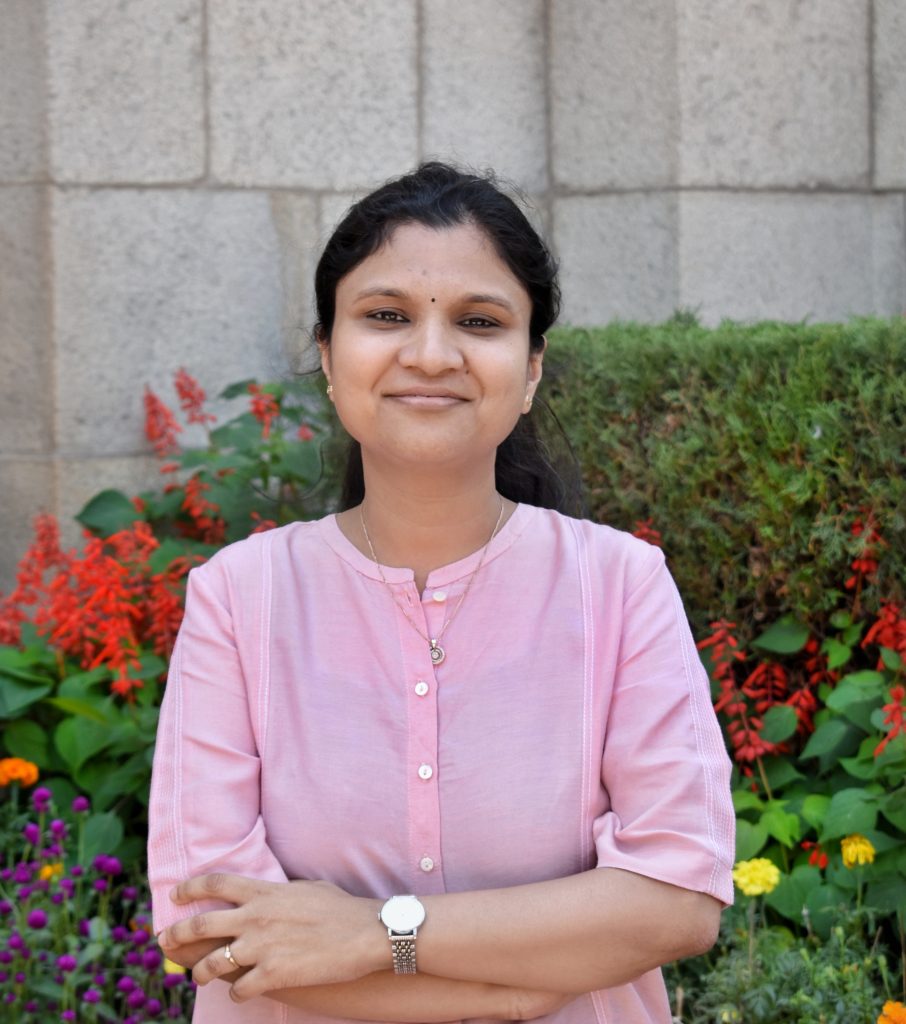
On a summer day in 2009, Garima Jindal was at IIT Bombay, waiting for her turn to attend the interview that would select her for a PhD programme. Born and raised in Delhi in a family of business owners, she had already bucked tradition by taking up science and pursuing chemistry for her Bachelor’s and Master’s studies. As she waited to be called, she caught whispers of a conversation among some of the other students and joined them. They were discussing a new and emerging field called computational chemistry. Intrigued, she sought out faculty member Raghavan B Sunoj’s lab, which specialised in this area, and joined his team. After completing her PhD and a postdoc, she joined IISc in March 2019 to establish her own lab at the Department of Organic Chemistry.
At IISc, her group, called the Computational Chemistry Lab, studies the mechanisms of catalytic reactions. These are chemical reactions that get a helping hand from catalysts. Imagine trying to climb a steep hill – it is energy-intensive. But a catalyst acts like a ramp, lowering the energy needed for the reaction to occur and making it happen faster.
The group studies two main types of catalysts: metals and enzymes. Metal catalysts are often transition metals like iron, copper, cobalt, platinum or palladium. They are commonly used in many industrial processes like making gasoline and hydrogenating vegetable oils. On the other hand, enzymes are natural biomolecules found in living organisms powering biological reactions such as digestion and respiration.
Garima’s research explores how these catalysts work on a molecular level by using computers to simulate reactions and understand the intricate steps involved. This is essential for designing better catalysts needed for cleaner and more efficient chemical processes.
“We study the role of a catalyst: how it interacts with the reactants and how it helps the reaction to proceed. By examining the reactants at the molecular level, we can generate an energy profile that reveals the energy barrier that the reactant must overcome to form an intermediate. This initial step allows us to identify the most likely reaction pathway (mechanism),” Garima explains.
To do this, her lab uses computational tools based on Molecular Dynamics (MD), Density Functional Theory (DFT), and quantum mechanics to understand the energy changes involved at each step in the reaction. Like choosing the best route for a trip, the reaction pathway with the lowest energy barrier is the most likely to happen. “The essence of a reaction lies in its mechanism,” she says. “Understanding the mechanism empowers us to strategically change the substrate or catalyst in synthesis experiments. By knowing how the molecule interacts with the catalyst, we can design new reactions with a much higher degree of control.”
Enzymatic catalysis is a rapidly growing field. Natural enzymes are incredibly efficient – reactions that they catalyse in mere seconds would take years in their absence. However, designing enzymes for artificial reactions in the lab or factory remains a challenge. While enzymes offer eco-friendly advantages like using water as a solvent, their sheer size and complexity (with tens of thousands of atoms) make it difficult to manipulate them experimentally. Our understanding of how enzymes achieve such remarkable efficiency is incomplete, preventing us from exploiting them fully in synthetic processes.
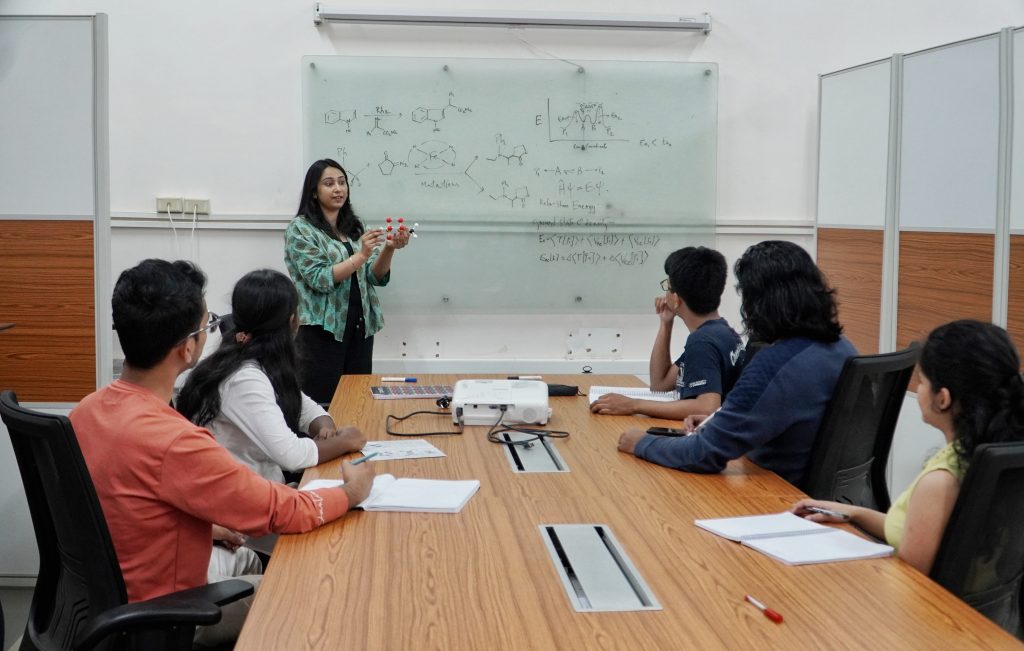
This is where computational tools come in. By dissecting the enzyme’s blueprint – its amino acid sequence – researchers can pinpoint the reaction site and identify the surrounding amino acid residues that play crucial roles in its role as a catalyst. “A single mutation – swapping one amino acid for another – can dramatically alter how well it works. Imagine an enzyme made of 300 amino acids, each with 20 possible replacements. This creates an enormous number of variations – far too many to test experimentally,” Garima elaborates. “Traditionally, scientists randomly changed amino acids and hoped for improvement. This inefficiency stemmed from a lack of understanding about how mutations affect enzymes. We are exploring how single mutations can affect the catalytic efficiency of enzymes. This can pave the way for a more systematic approach to engineering enzymes with desired properties.”
Studying enzymes, with their massive size (20,000-30,000 atoms) compared to transition metal catalysts (300-400 atoms), presents a unique challenge computationally. While traditional quantum mechanics works well for transition metal catalysts, scientists use molecular dynamics (MD) simulations to study enzymes. Enzymes constantly move and fluctuate with time, existing in a range of conformations. Crystal structures provide a static snapshot of an enzyme, but they don’t capture this movement when the enzyme is surrounded by a solvent. MD simulates how an enzyme moves over time, allowing researchers to see how different parts fluctuate and interact with each other and the solvent. This information is crucial in understanding enzyme efficiency. By using MD simulations alongside crystal structures, researchers can build a more complete picture of enzyme behaviour and design new enzymes.
“Our research group has made significant strides. We developed a compelling transition state model for a metal-catalysed reaction, a feat difficult to achieve through experiments alone. Supporting DFT calculations and phosphorus NMR have given us a much deeper understanding of the model,” Garima says. Her lab uses NMR analysis and experiments to validate the predictions from the computer simulations.
Garima’s group also plans to develop expertise in kinetics studies, which probe reaction rates and provide insights into reaction mechanisms that complement DFT calculations. Recent developments in AI and ML are finding use in reaction design as well. “This data-driven approach is particularly useful when the mechanism is unknown. They could be used to identify key features of reactants, catalysts and products from successful reactions reported in literature, informing the design of even better ones. Scientists can even combine both strategies – a general understanding of the mechanism with AI/ML tools – to refine reaction design,” says Garima.
Staying on top of the 20 or so ongoing projects she has keeps Garima quite busy. Her lab’s recent forays into wet lab experiments have been an additional challenge for a computational chemist like her.
She also strives to ensure she keeps track of her students’ progress, she says. “I have this constant worry: Am I letting them down? Did I provide them the right projects or guidance? Slowly, I have learnt to trust my students to take ownership of their research while I guide and support them. I tell them that research is a marathon not a sprint.” Some new students come to her with a fear for programming or modelling, she says. She reassures them that all they need is a curious and open mind to succeed in the field.
Computational chemistry is a fast-growing field, Garima emphasises. “I want computational and experimental chemists to collaborate on catalyst design and development to achieve the Holy Grail: designing novel catalysts with targeted properties. That is the future that I would like to see in India and globally.”
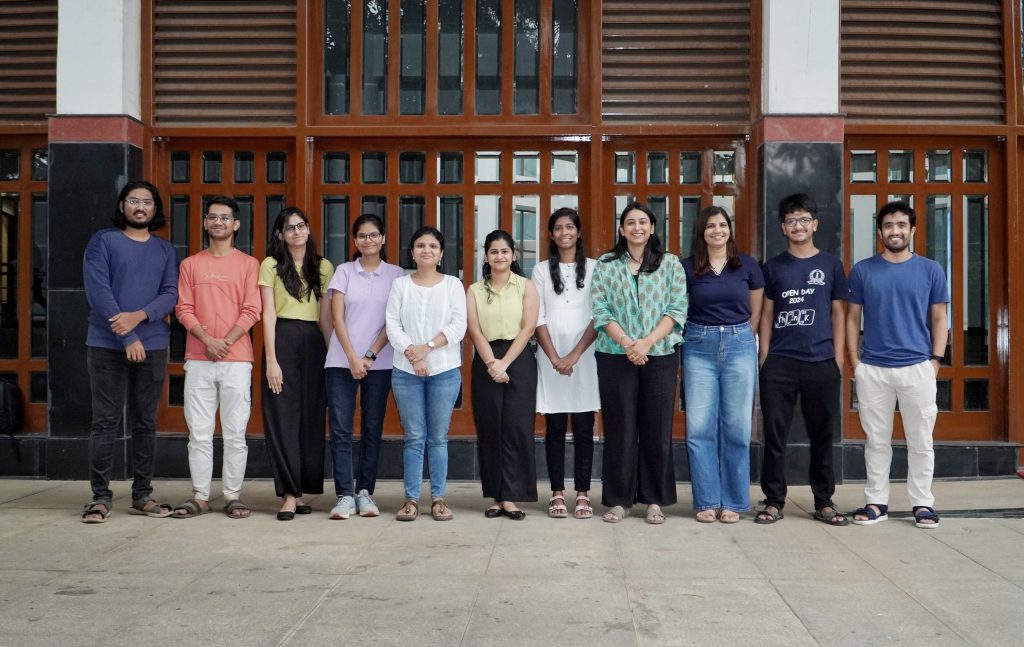