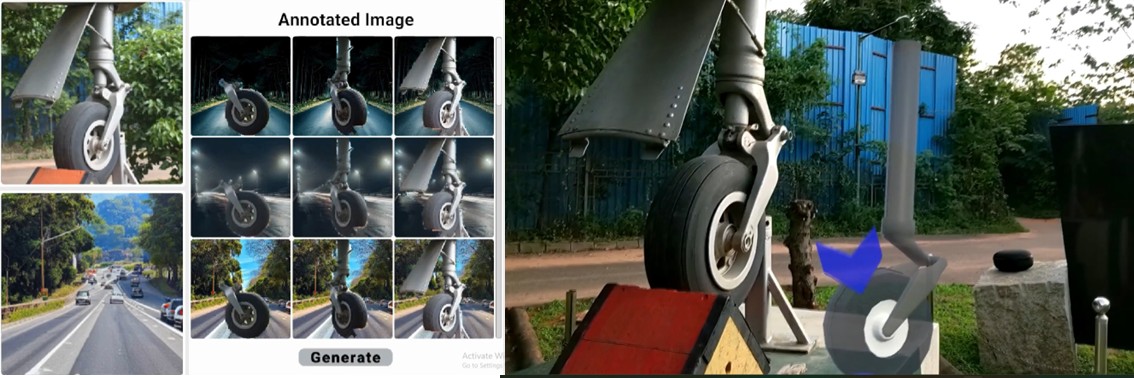
In recent years, Mixed Reality (MR) technologies – where digital and physical elements are blended – are increasingly finding applications in manufacturing and maintenance industries. These systems often rely on artificial intelligence (AI) to identify and interact with real-world objects. However, to train these AI models effectively, a large and diverse collection of images is needed. Gathering such real-world data can be expensive, time-consuming, and sometimes impossible in industrial settings due to safety or access restrictions.
Researchers at the Department of Design and Manufacturing (DM), IISc led by Pradipta Biswas have found a creative solution: synthetic image generation. Instead of collecting thousands of real photographs, they used a special kind of artificial intelligence approach, called a diffusion model, to generate realistic images. They took images of real objects – such as parts of a pneumatic cylinder – and blended them with different background scenes where object detection has previously struggled. This helps the model “see” the object in a wide variety of settings, making it better at recognising the object in real life.
The team tested this method against two other common techniques: traditional editing and GAN. The diffusion-based approach led to much higher accuracy in detecting objects, even though it used fewer images. Specifically, it improved the detection performance by 11% while using 67% fewer images than the traditional methods. Additionally, the team has created an easy-to-use interface so that others can generate their own synthetic data without deep technical knowledge. This makes it a powerful tool for improving machine learning models in MR applications where data is limited.
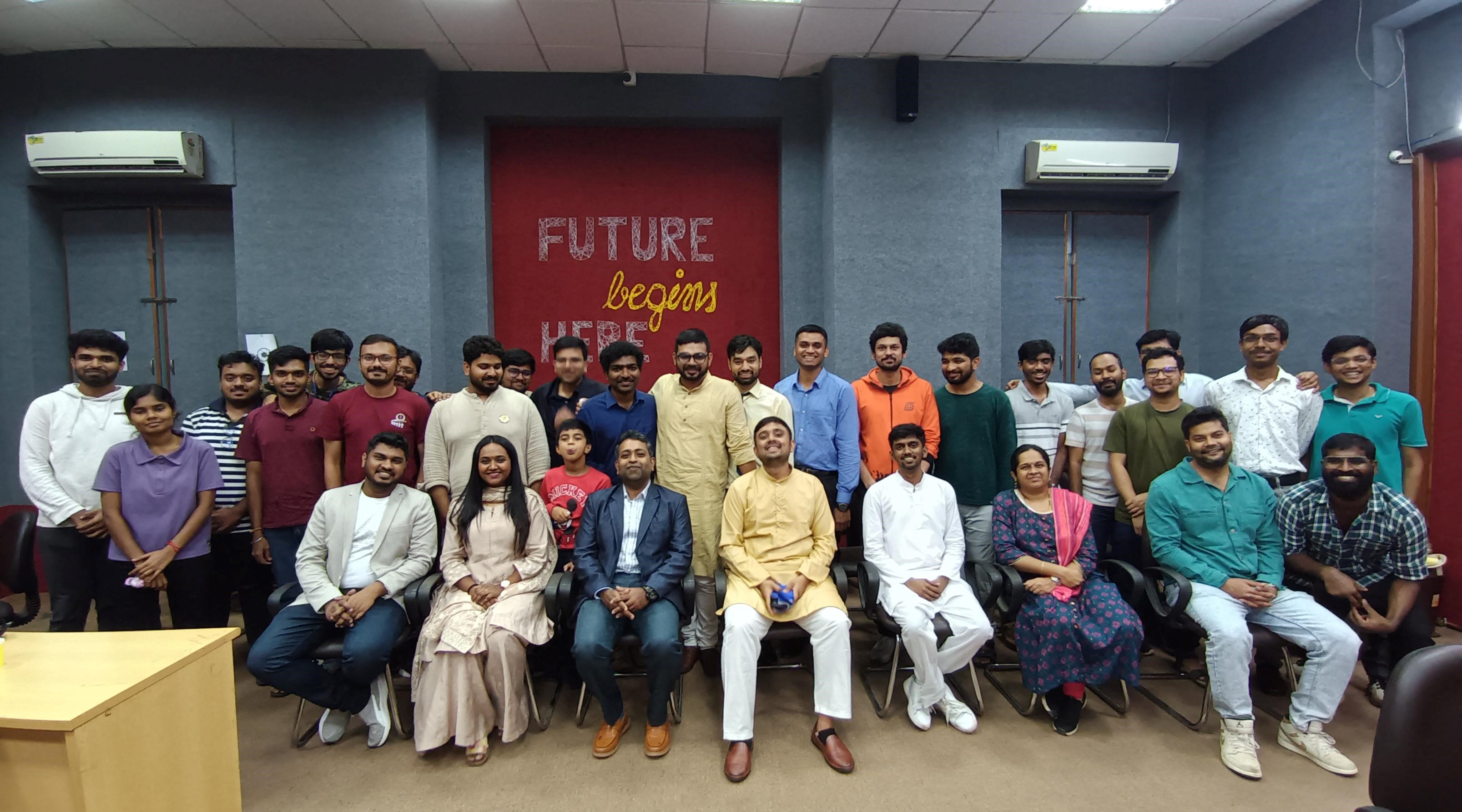