New initiatives aim to get AI to understand Indian languages and accents
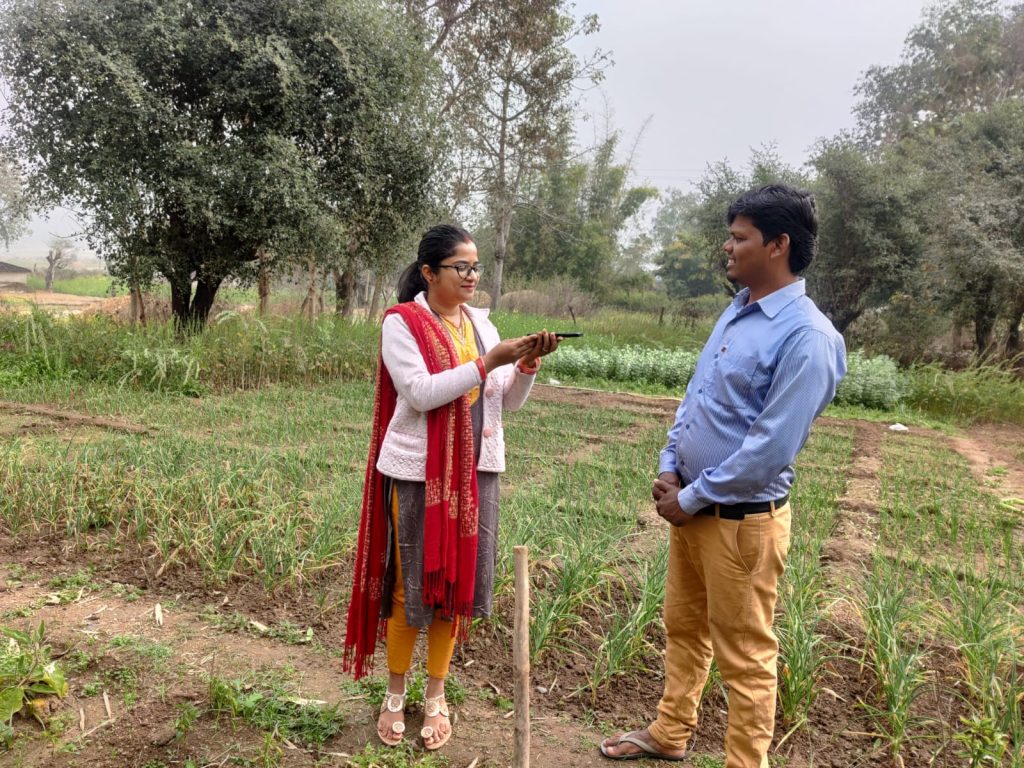
Many businesses today employ Artificial Intelligence (AI)-based customer services. These use automated messages that ask customers to verbalise their problem (“Please state your query”). But the user often finds this a frustrating experience, since it takes multiple attempts for the machine or computer model imitating a person to understand the user, invariably leading to nostalgia for the times when one could directly speak to a human being.
Though flawed, the technology of voice recognition is meant to make day-to-day life easier, not just for the urban population but also for rural dwellers. In many parts of rural India, people must travel far distances merely to deposit a cheque in a bank, or to find information about new pesticides and fertilisers. Even finding timely medical help can be a luxury. The recent pandemic has underscored the need for such speedy services even more.
According to a 2022 report, at least 98% of Indians have mobile coverage, and each of them can, in principle, use it to search for information about their daily needs and perform simple transactions. But there is a problem. Even assuming that the devices are becoming affordable every day, that network connectivity is powerful and that there is 24-hour supply of electricity, to take full advantage of this technology, the user must be able to speak, read and write English.
Unfortunately, barely 10% of Indians are conversant in English, write Prasanta Kumar Ghosh, Associate Professor at the Department of Electrical Engineering (EE), IISc, and Raghu Dharmaraju, President, ARTPARK, IISc, in a blogpost. This means that almost a billion Indians are excluded from availing assistive technologies. In an ideal world, all of us should be able to ask questions in our own language, in our own accent, and get reliable, error-free answers. “That is why an inclusive Digital India requires language AI that can understand all Indians,” they write.
The limiting factor is the availability of sufficient data to develop speech-based technologies. To overcome this challenge, three major speech-related projects were initiated at IISc in the last three years, under the guidance of Prasanta, who has worked in speech processing for almost 20 years, with collaborators at IISc and ARTPARK. The projects are RESPIN, funded by the Bill and Melinda Gates Foundation, which started in May 2021 to collect speech samples for converting speech to text; SYSPIN, funded by the German Development Cooperation, which started in July 2021 to convert text to speech; and finally, VAANI, a project that started in December 2022, is funded by Google, and seeks to collect speech samples from every district in India.
Searching for speech
The idea is to train a computer or machine learning model to recognise inflections in Indian languages using a large set of examples from native speakers. Prasanta says that Indian language datasets are not openly and easily available, and some agencies outside the country make such datasets available for purchase. “So, if I want one, I have to purchase it from them for thousands of Euros.” He finds this demoralising as he believes that we ourselves have the capacity to collect such data. “It is our responsibility to do something for the speech community in India, for speech technology development in Indian languages.” Which is why Prasanta, who claims he is fervently against “colonising data”, readily joined hands with the Gates Foundation, whose mandate is to make all the RESPIN data open source.
RESPIN focuses on speech recognition in the domains of agriculture and finance, the two pillars of development for any country, according to the Gates Foundation. The data is to be collected specifically from rural areas where people are often illiterate or economically backward.
Sandhya Badiger, data manager and one of the earliest recruits at RESPIN, says that the data is being collected in nine languages – five major (Hindi, Bengali, Marathi, Kannada and Telugu) and four low-resourced ones (Bhojpuri, Maithili, Magahi and Chhattisgarhi). There are 3-5 dialects for each, and the target is to collect about 1,000 hours of speech samples from 2,000 native speakers in each language, divided equally between these dialects. The IISc team partners with companies like NAVANA Tech, who go out into the field to collect voice samples. The way they do this is by asking the speakers to read aloud specifically designed sentences related to agriculture and finance. This data is then curated at IISc by engineers like Sandhya, Saurabh Kumar and Sathvik Udupa, Research Associates working on RESPIN, who then use it to train different types of machine learning models to convert speech to text.
But this is easier said than done. Sandhya gives an example of a dialect spoken in Kishanganj district on the Bihar-West Bengal border. The older generation there has a better hold on this endangered language, but many of them are illiterate. The younger ones, who can read, often have Hindi or Bhojpuri influence in their speech. “We must choose the speakers carefully,” she says, “and develop methods to nullify such problems.”
During the second phase of RESPIN, the team plans to build models based on the collected samples and test them in the field to see if the models can take speech as input and give out error-free text as output. “Right now [during the data collection phase], the speakers are reading out sentences that we give them. In the field, they are not going to do that, rather they will speak spontaneously,” says Prasanta. Success will depend upon how well the model is able to look beyond the speaker’s age, gender, educational background, and the influence of other languages they speak on their speech. In addition, since the model is trained to recognise specific terminology, the programmes that are developed for agriculture and finance may not work in other areas.
The second project, SYSPIN, also uses the same nine languages, but there, the goal is reverse: converting text to speech. The model is trained using voice samples from only two speakers – one male and one female – from each language. The chosen speakers are expert voice artists, and the recordings – about 50 hours each – are of very high quality. The model is trained to mimic these voices and speak like them.
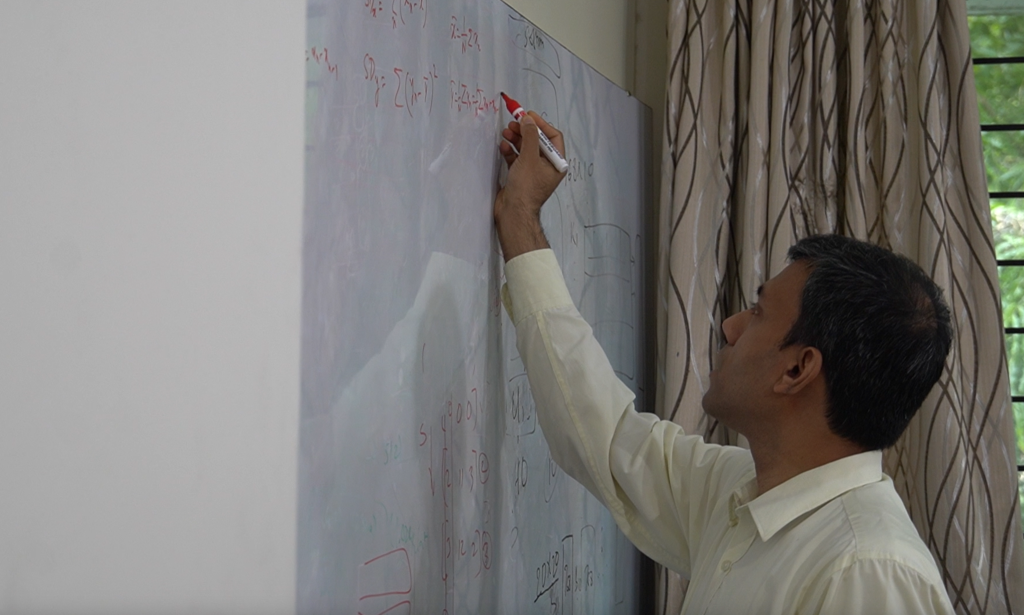
A language colour wheel
While Prasanta and his team were working on these two projects, Google, which had its own 1,000 languages AI initiative, was keen to build Indian language speech resources. “They approached us for all the languages in India, not just nine,” says Prasanta.
Prasanta likens the diversity of Indian languages to a continuous colour wheel. Just as adjacent colours merge with each other, and there are several shades of yellow, red, or blue, so do languages have both, variety and overlap. This is why he believes that to get a real “map” of all Indian languages, data must be collected from as many speakers in as many areas as possible. VAANI, the project funded by Google, aims to collect 200 hours of speech data from every single district in India. It will be stored according to pin codes and will be made public, Prasanta explains.
In RESPIN, samples are collected from speakers who read out sentences given to them; in VAANI the speakers will describe a set of images in the language they speak at home. “Speakers may speak in Ahirani, Angika or Nalgonda style Telugu. They give us the name of their languages, and we record it as such,” Prasanta says. Data from 80 districts have been targeted in the first phase of VAANI.
Prasanta points out that there are several practical challenges for a project like this. “How do I create data for all the people? Should I take the same sentence/image and get all 1.3 billion Indians to say it? How many sentences/images should I use? To get good data, how should I start? How many speakers should I ask? What (images) do I ask them to describe? In what context? Under what conditions? There are no [clear] answers to many of these questions.”
The team also conducts “challenges” to validate their data. For example, at a recent conference, they gave 40 hours of voice recordings – of a male and a female speaker – in Hindi, Marathi and Telugu, and told the participants to build their best text-to-speech programme. Companies like Microsoft, as well as some Indian and Chinese organisations participated. At an upcoming conference in Taiwan, 40 teams will work on the Bengali and Bhojpuri language corpora. “Such challenges give visibility to the project and Indian language data. Imagine someone from China working on Marathi!” exclaims Prasanta. What’s interesting is that the programmer does not need to know the language; only the sound of the sentences, which is then converted by a trained computer to the native script.
Prasanta expects that such data, when collected, will be useful not just to the developers of speech-based technologies, but also to linguists, conservators of endangered languages, and those who want to create content in languages that have no scripts. Once the enormous variety and shades of Indian languages are preserved in the form of clean data, the possibilities are limitless.