Successful change detection may be linked to how some people are better at selectively focusing on specific objects
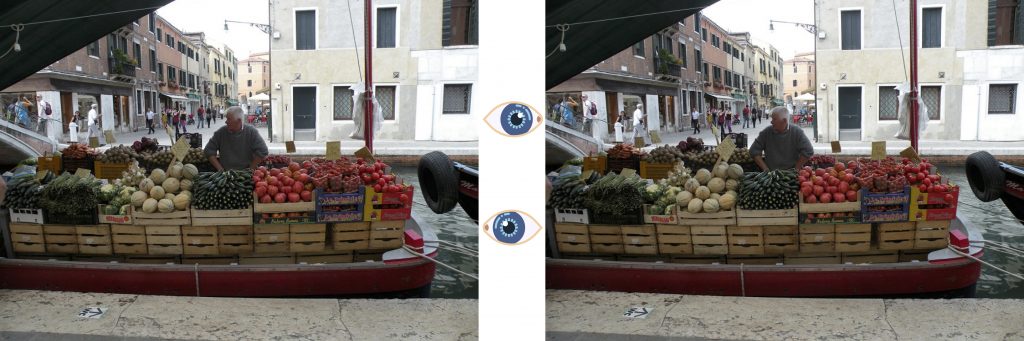
Can you spot the difference in the two images above?
Our brains have the remarkable ability to pay attention to details but may sometimes fail to notice even marked differences. This phenomenon of overlooking a visual change, or ‘change blindness’, has been studied by a research group at the Centre for Neuroscience (CNS) and the Department of Computer Science and Automation (CSA). They have developed a novel computational model of eye movement that can predict a person’s ability to detect changes in their visual environment. The researchers believe that successful change detection may be linked to enhanced visual attention – how some people are better at selectively focusing on specific objects.
In the study, the team first checked for change blindness among 39 people by showing them an alternately flashing pair of images that have a minor difference between them. In the above image, for example, the difference lies in the size of the tyres on the extreme right.
“We expected some complex differences in eye movement patterns between subjects who could do the task well and those who could not. Instead, we found some very simple gaze-metrics that could predict the success of change detection,” recounts Sridharan Devarajan, Associate Professor at CNS, and corresponding author of the paper. Successful change detection was found to be linked to two metrics: how long the subjects’ gaze was fixated at a point, and the variability in the path taken by their gaze between two specific points (‘saccade amplitude’). Subjects who fixated for longer at a particular spot, and whose eye movements were less variable were found to detect changes more effectively.
Based on these observations, the researchers developed a computational model that can predict how well a person might be able to detect changes in a sequence of similar images shown to them. The model also takes into consideration various biological parameters, constraints and human bias. “Since biological neurons are ‘noisy’, they do not encode the image precisely,” Sridharan explains. He adds that there is a lot of variability in the way neurons encode – process and/or respond to – images in the brain, which can be captured by a mathematical representation called the Poisson process.
Other researchers have previously developed models that focus either only on eye movement or on change detection, but the model developed by the IISc team goes one step further and combines both.
The researchers also tested their model against a state-of-the-art deep neural network called DeepGaze II, and found that their model performed better at predicting human gaze patterns in free-viewing conditions – when the subjects were casually viewing the images. While DeepGaze II could predict where a person will look if presented with an image, it did not work as well as the IISc-developed model at predicting the eye movement pattern of a person searching for a difference in the images.
In the future, the researchers also plan to incorporate artificial neural networks with “memory” into the model – to more realistically mimic the way our brains retain recollections of past events to detect changes.
The authors say that the insights into understanding change blindness provided by their model could help scientists better understand visual attention and its limitations. Some examples of areas where such insights can be applied include diagnosing neurodevelopmental disorders like autism, improving road safety while driving or enhancing the reliability of eyewitness testimonies.